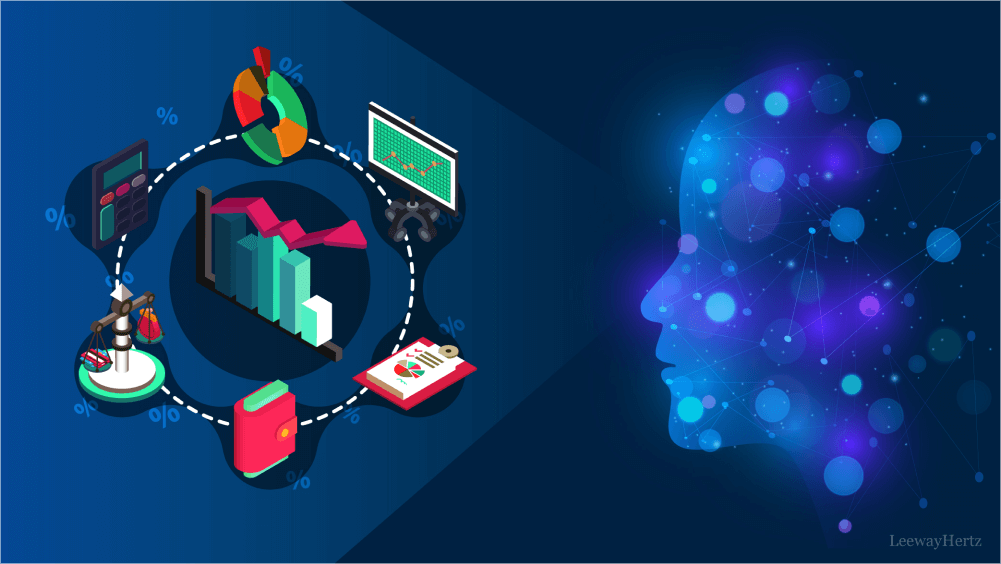
Financial planning and forecasting are critical components of effective business management. Accurate predictions and strategic planning can make the difference between success and failure. With the advent of ai-powered analytics, businesses can now leverage deeper insights and make more informed financial decisions. This blog explores how ai-driven insights enhance financial planning and forecasting.
The role of ai in financial planning and forecasting
Ai leverages advanced algorithms, machine learning, and data analytics to process vast amounts of financial data, identify patterns, and predict future trends. By automating complex calculations and providing real-time analysis, ai-driven tools enable businesses to enhance the accuracy and efficiency of their financial planning processes.
Key benefits of ai-powered financial planning and forecasting
1. Enhanced accuracy and precision
Key points:
Data-driven predictions: ai uses historical data and real-time information to generate highly accurate forecasts.
Error reduction: automated calculations reduce the risk of human errors, ensuring more reliable financial projections.
Example: a manufacturing company uses ai to forecast demand for its products, resulting in more accurate production planning and inventory management.
Statistical insight: according to gartner, ai-driven financial planning can improve forecast accuracy by up to 50%.
2. Real-time data analysis
Key points:
Continuous monitoring: ai systems continuously analyze financial data, providing up-to-date insights and identifying trends as they develop.
Timely adjustments: real-time analysis allows businesses to make timely adjustments to their financial strategies based on current data.
Example: a retail chain uses ai to monitor sales data in real-time, adjusting marketing and inventory strategies based on emerging trends.
Statistical insight: mckinsey reports that real-time data analysis can enhance financial decision-making speed by 30%.
3. Predictive analytics
Key points:
Trend identification: ai identifies patterns and trends that may not be immediately apparent through traditional analysis methods.
Future scenarios: predictive analytics models simulate various future scenarios, helping businesses prepare for different outcomes.
Example: an e-commerce company leverages ai-driven predictive analytics to forecast seasonal sales trends, optimizing its promotional campaigns and stock levels.
Statistical insight: according to accenture, companies using predictive analytics see a 20% improvement in financial forecasting accuracy.
4. Comprehensive risk management
Key points:
Risk detection: ai identifies potential financial risks by analyzing patterns and anomalies in financial data.
Proactive mitigation: businesses can proactively address identified risks, minimizing their impact on financial performance.
Example: a financial services firm uses ai to detect early signs of market volatility and adjust its investment strategies accordingly.
Statistical insight: a study by deloitte found that ai-driven risk management can reduce financial losses from unforeseen risks by 30%.
5. Enhanced strategic planning
Key points:
Scenario planning: ai enables businesses to create detailed financial models for various strategic scenarios.
Resource allocation: improved insights help businesses allocate resources more effectively, optimizing performance and growth.
Example: a tech startup uses ai-driven scenario planning to explore different growth strategies, deciding where to allocate funds for maximum impact.
Statistical insight: according to pwc, ai-powered strategic planning can improve resource allocation efficiency by 25%.
Implementing ai-driven financial planning and forecasting
1. Selecting the right ai tools
Key points:
Feature set: choose ai tools that offer comprehensive analytics, predictive modeling, and real-time data processing capabilities.
Integration: ensure the tools can integrate seamlessly with your existing financial systems and data sources.
Example: a company selects an ai-powered financial planning tool that integrates with its erp system and provides real-time forecasting and scenario planning features.
2. Data preparation and management
Key points:
Data quality: ensure your data is accurate, complete, and up-to-date to maximize the effectiveness of ai analysis.
Data integration: consolidate data from various sources into a unified system for comprehensive analysis.
Example: a retail business cleans and consolidates its sales, inventory, and customer data before feeding it into the ai system for analysis.
3. Training and support
Key points:
Employee training: provide training to ensure your team understands how to use ai tools effectively and interpret the insights generated.
Ongoing support: offer continuous support to address any issues and optimize the use of ai tools.
Example: an accounting firm conducts workshops to train its financial analysts on using ai-powered forecasting tools and interpreting the results.
4. Continuous monitoring and optimization
Key points:
Performance tracking: regularly monitor the performance of ai tools and the accuracy of their predictions.
Feedback loop: establish a feedback loop to gather insights from users and continuously improve the ai models.
Example: a manufacturing company continuously tracks the performance of its ai-driven demand forecasts, using the insights to refine and enhance the models.
Conclusion
Ai-driven financial planning and forecasting offer significant benefits, including enhanced accuracy, real-time data analysis, predictive insights, comprehensive risk management, and improved strategic planning. By selecting the right ai tools, ensuring data quality, providing proper training, and continuously monitoring performance, businesses can leverage ai to transform their financial planning processes and achieve better outcomes.